
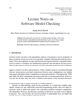
Directed evolution is a widely-used strategy of protein engineering to improve protein function via mimicking natural mutation and selection. Machine learning-assisted directed evolution (MLDE) approaches aim to learn a fitness predictor, thereby efficiently searching for optimal mutants within the vast combinatorial mutation space. Since annotating mutants is both costly and labor-intensive, how to efficiently sample and utilize informative protein mutants to train the predictor is a critical problem in MLDE. Previous MLDE works just simply utilized pre-trained protein language models (PPLMs) for sampling without tailoring to the specific target protein of interest, which has not fully exploited the potential of PPLMs. In this work, we propose a novel method, the Actively-Finetuned Protein language model for Directed Evolution(AFP-DE), which leverages PPLMs to actively sample and fine-tune themselves, continuously improving the model’s sampling and overall performance through iterations, to achieve efficient directed protein evolution. Extensive experiments have shown the effectiveness of our method in generating optimal mutants with minimal annotation effort, outperforming previous works even with fewer annotated mutants, making it budget-friendly for biological experiments.