
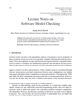
Semantic text matching has a wide range of applications in natural language processing. Recently proposed models that have achieved excellent results on short text matching tasks are not well suited to long-form text matching problems due to input length limitations and increased noise. On the other hand, long-form texts contain a large amount of information at different granularities after encoding, which cannot be fully interacted and utilized by existing methods. To address above issues, we propose a novel long-form text-matching framework which fuses Bi-Encoder and Cross-Encoder (FBC). Specially, it first employs an entity-driven key sentence extraction method to obtain the crucial content of the text and filter out noise. Subsequently, it integrates Bi-Encoder and Cross-Encoder to better capture semantic features and matching signals. Extensive experiments on several publicly available datasets demonstrate the effectiveness of our approach, compared with strong baselines. Furthermore, our model exhibits greater stability and accuracy in determining the matching relationship between documents describing the same event, which outperforms previously established approaches. The code is released at https://github.com/CSU-NLP-Group/FBC.