
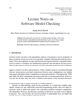
Unsupervised Video Anomaly Detection (UVAD) utilizes completely unlabeled videos for training without any human intervention. Due to the existence of unlabeled abnormal videos in the training data, the performance of UVAD has a large gap compared with semi-supervised VAD, which only uses normal videos for training. To address the problem of insufficient ability of the existing UVAD methods to learn normality and reduce the negative impact of abnormal events, this paper proposes a novel Enhanced Spatio-temporal Self-selective Learning (ESSL) framework for UVAD. This framework is designed for capturing both the appearance and motion features through effective network structures by solving the spatial and temporal jigsaw puzzles. Specially, we develop a Self-selective Learning Module (SLM) for UVAD, which prevents the model learning abnormal features and enhances the model by selecting normal features. Experimental results on three benchmark datasets show that the proposed method not only surpasses the state-of-the-art UVAD works, but also achieves the performance comparable to the classic semi-supervised methods for video anomaly detection that needs normal videos selected manually. Code is available at: https://github.com/xusuger/ESSL.