
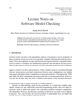
Existing deep subspace clustering algorithms are difficult to scale to large-scale data. There are two reasons: Firstly, the existing subspace clustering algorithms almost all need to find the self-expressive coefficient matrix whose size is proportional to the square of the data set size at once. Secondly, spectral clustering needs to solve the eigenvector of the affinity matrix. These two points make the computational complexity of clustering very high when the data scale is large. This paper proposes Self-Expressive Network-Based Deep Embedded Subspace Clustering (SE-DESC), a subspace clustering method that can be applied to large-scale single-view and multi-view data. Using the idea of siamese networks, we design a self-expressive network to calculate the self-expressive coefficient between two data points, reducing the parameter amount of the self-expressive model to a constant. It can effectively avoid computational complexity. Then, we use a deeply embedded network to learn an embedding for each data point to map the data into the spectral space, avoiding the high computational complexity of spectral clustering. Extensive experiments demonstrate that SE-DESC improves the clustering performance on large-scale data compared to state-of-the-art methods.