
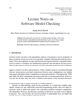
Stories about everyday situations are an essential part of human communication, motivating the need to develop AI agents that can reliably understand these stories. Despite the long list of supervised methods for story completion and procedural understanding, current AI fails to generalize its procedural reasoning to unseen stories. This paper is based on the hypothesis that the generalization can be improved by associating downstream prediction with fine-grained modeling and the abstraction of procedural knowledge in stories. To test this hypothesis, we design LEAP: a comprehensive framework that reasons over stories by jointly considering their (1) overall plausibility, (2) conflict sentence pairs, and (3) participant physical states. LEAP integrates state-of-the-art modeling architectures, training regimes, and augmentation strategies based on natural and synthetic stories. To address the lack of densely annotated training data on participants and their physical states, we devise a robust automatic labeler based on semantic parsing and few-shot prompting with large language models. Our experiments with in- and out-of-domain tasks reveal insights into the interplay of architectures, training regimes, and augmentation strategies. LEAP’s labeler consistently improves performance on out-of-domain datasets, while our case studies show that the dense annotation supports explainability.