
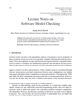
The opaqueness of deep neural networks hinders their employment in safety-critical applications. This issue is driving the research community to focus on eXplainable Artificial Intelligence (XAI) techniques. XAI algorithms can be categorized into two types: those that explain the predictions of black-box models and those that create interpretable models from the start. While interpretable models foster user trust, their performance is normally inferior to traditional black-box models like neural networks. To fill this gap, this chapter presents an extensive framework introducing special neural networks known as Logic Explained Networks (LENs). The most notable advantage of this approach is that LENs achieve performances that are comparable to the state-of-the-art neural networks while providing concise First-Order Logic explanations. Moreover, LENs are designed to learn various types of explanations, such as local or global, by associating related task functions or directly explaining a single class based on the others and/or the input data. They can offer explanations for their behaviour or that of other black-box models. Finally, LENs can be applied in many learning scenarios: they can be trained using supervised or unsupervised learning and applied across different domains like tabular data, computer vision, natural language processing, and relational tasks.