
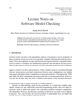
Strict demands for very tight tolerances and increasing complexity in the semiconductors’ assembly impose a need for an accurate parametric design that deals with multiple conflicting requirements. This paper presents application of the advanced optimization methodology, based on evolutionary algorithms (EAs), on two studies addressing parametric optimization of the wire bonding process in the semiconductors’ assembly. The methodology involves statistical pre-processing of the experimental data, followed by an accurate process modeling by artificial neural networks (ANNs). Using the neural model, the process parameters are optimized by four metaheuristics: the two most commonly used algorithms – genetic algorithm (GA) and simulated annealing (SA), and the two newly designed algorithms that have been rarely utilized in semiconductor assembly optimizations – teaching-learning based optimization (TLBO) and Jaya algorithm. The four algorithm performances in two wire bonding studies are benchmarked, considering the accuracy of the obtained solutions and the convergence rate. In addition, influence of the algorithm hyper-parameters on the algorithms effectiveness is rigorously discussed, and the directions for the algorithm selection and settings are suggested. The results from two studies clearly indicate superiority of the TLBO and Jaya algorithms over GA and SA, especially in terms of the solution accuracy and the built-in algorithm robustness. Furthermore, the proposed evolutionary computing-based optimization methodology significantly outperforms the four frequently used methods from the literature, explicitly demonstrating effectiveness and accuracy in locating global optimum for delicate optimization problems.