
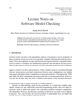
Point clouds are currently used for a variety of applications, such as detection tasks in medical and geological domains. Intelligent analysis of point clouds is considered a highly computationally demanding and challenging task, especially the segmentation task among the points. Although numerous deep learning models have recently been proposed to segment point cloud data, there is no clear instruction of which exactly neural network to utilize and then incorporate into a system dealing with point cloud segmentation analysis. Besides, the majority of the developed models emphasize more on the accuracy rather than the efficiency, in order to achieve great results. Consequently, the training, validation and testing phases of the models require a great number of processing hours and a huge amount of memory. These high computational requirements are commonly difficult to deal with for many users. In this article, we analyse five state-of-the-art deep learning models for part segmentation task and give meaningful insights into the utilization of each one. We advance guidelines based on different properties, considering both learning-related metrics, such as accuracy, and system-related metrics, such as run time and memory footprint. We further propose and analyse generalized performance metrics, which facilitate the model evaluation phase in segmentation tasks allowing users to select the most appropriate approach for their context in terms of accuracy and efficiency.