
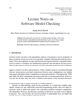
Applications that process Point-of-Interest data are omnipresent nowadays. They range from digital maps to recommender systems for places to visit, and personal assistants. The success of such applications critically depends on the quality of the ingested data. However, corresponding databases, especially when they are crowdsourced, are often incomplete. Existing work on automatic data completion approaches has only partially considered the task for Points-of-Interest (POI). Such entities have a number of distinctive properties – notably multiscript names, geo-spatial identity, and temporally defined context –, which make the task more challenging. Here we present an approach to automatically complete POI semantic tags in a crowdsourced database. We perform experiments on multi-lingual data from Foursquare, a global location-based social network, and observe that (i) POI names are strong predictors of POI semantic tags: a character-based LSTM model trained only on POI names gives 72.5% worst-case micro-precision and 50.38% micro F1 scores, (ii) appropriate use of spatio-temporal data leads to consistent improvements (iii) using a structured representation of time gives higher precision and requires less computation time than string-based LSTM variants, however, the higher precision is achieved at a cost of lower recall and micro-F1, (iv) an LSTM model trained on semi-structured strings representing time, is competitive to fully structured inputs in terms of recall.