
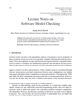
We study the indoor positioning problem by using multiple beacons inside a building and transforming the problem into a binary classification problem in which a person is detected if he or she is working nearby one beacon within a radius d > 0. By designing our own beacons and implementing a mobile application that can communicate with each beacon via Bluetooth Low Energy (BLE), we extract meaningful features from signals of beacons during a time window and generate training and testing datasets in order to learn an appropriate classifier which can achieve as high performance as possible. We consider six classification methods including linear SVM, logistic regression, random forest, decision tree, XGBoost, and neural networks for training the binary classification model and measuring the corresponding performance by precision, recall, F1-score, and AUC. The experimental results show that using random forest with a distance pre-filter can obtain the highest performance in terms of both F1-score and AUC. The outcome of the paper can give an additional contribution to the indoor positioning problem, especially in the inspection industry where the proposed algorithms can be applied for verifying if an inspector is working in the right factory and nearby important places inside the factory. It can also reduce the risk of fraudulent behaviors in important inspections.