
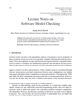
Accurate air quality prediction is crucial for environmental monitoring and public health. This study explores a novel approach using machine learning algorithms and large language models to predict the Air Quality Index (AQI). While traditional AQI prediction relies on complex models and extensive data on pollutants and meteorological factors, this research investigates the use of readily available fuel consumption data as an alternative predictor, given its close link to emissions. The study employs supervised machine learning algorithms, including Random Forest and Gradient Boosting, utilizing fuel consumption data as input features to build predictive models. Additionally, a state-of-the-art large language model, GPT-3.5-turbo-instruct, is fine-tuned on historical AQI data and evaluated for its predictive capabilities. The performance of both machine learning models and the language model is compared using various metrics, and the results demonstrate that both approaches achieve high AQI prediction accuracy, outperforming traditional methods based on pollutant concentration data. Notably, the fine-tuned language model exhibits superior performance, potentially due to its ability to capture complex dependencies and contextual information from the training data. This work highlights the potential of leveraging readily available fuel consumption data and advanced language models for accurate and cost-effective AQI prediction. The findings have significant implications for developing scalable air quality monitoring systems, enabling timely interventions and informed decision-making to mitigate the adverse effects of air pollution.