
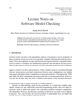
Traffic sign detection (TSD) is a significant task in the field of computer vision, which has important applications in traffic safety and driverless driving. However, this fundamental but challenging task still has always influenced by numerous negative factors, such as light intensity, severe weather and distance. In addition, this task is characterized by the small scale and irregular distribution of detected objects in complex traffic scenes. To mitigate these aforementioned challenges, this paper presents a method for TSD task improved on YOLOv5 with specific improvements. First, to alleviate the adverse impact posed by noise and enhance the feature representation, we design an adaptive network to preprocess the input image. Subsequently, we introduce recursive gated convolution and a second-order attention module in the neck to improve the sensibility of the proposed structure for small objects, i.e., traffic signs in the large-scale and complex scene. Finally, Control Distance Intersection over Union (CDIoU) loss is utilized to accelerate the convergence progress while ensuring the model detection effect. Experimental results illustrate qualitatively and quantitatively that our method outperforms other state-of-the-art detection methods in TSD.