
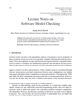
One of the critical indicators for assessing the practical applicability of large language models is their competency in vertical domain question-answering tasks. However, in real-world applications, fine-tuning these large models often compromises their inherent capabilities. Moreover, fine-tuning does not offer precise control over the model’s generated outputs.Consequently, enhancing the question-answering performance of large models in specialized domains has become a focal concern in the field. To address these challenges, this paper introduces a novel approach for generating and optimizing a “Chain-of-Thought”(CoT), leveraging domain-specific knowledge graphs. Specifically, we propose a Knowledge Graph-generated Chain of Thought (KGCoT) method that utilizes graph search algorithms to generate a chain of thought. This chain guides the injection of specialized knowledge into large language models and adapts the weightings based on user feedback, thereby optimizing subsequent graph searches.Heuristic searches are performed on the knowledge graph based on edge weights, culminating in the amalgamation of discovered entities and knowledge into a chain of thought. This KGCoT serves as a prompt to stimulate the large language model’s contemplation of domain-specific knowledge. Additionally, an adaptive weight optimization formula refines the chain’s weights in response to output feedback, thereby continually enhancing the quality of future search results and ensuring real-time optimization capabilities for the model.Through empirical evaluations conducted on publicly available datasets, the large language model ChatGLM, when prompted with a KGCoT, exhibited a 72.8% improvement in its BLEU score compared to its baseline performance. This outperformed other models like LLaMA and RWKV, unequivocally substantiating the efficacy of the proposed KGCoT method.