
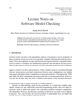
Digital media technology plays an important role in helping people to better access, disseminate and share information, promote people’s communication and interaction, and promote the development and progress of society. Image classification is one of the important technologies of digital media. It is of great significance to improve the development and progress of image classification algorithm in digital media technology. However, the existing image classification algorithms generally have the problem of insufficient flexibility or accuracy. Based on this, an image classification algorithm based on YOLO technology is proposed in this paper. In order to reduce the training cost of the model, the standard convolutional module and feature extraction network in the original model are replaced by a depth separable convolutional module and a lightweight network MobileNetV2. Secondly, an image preprocessing method is proposed to locate and cut out the workpiece area in the image before input detection network. Then, a new data enhancement method was introduced to avoid the poor performance of the original mosaic data enhancement method in this data set and to prevent the over-fitting of training. In order to accelerate the training convergence, the model was pre-trained by transfer learning. Finally, the reliability of our model is verified on the real data set.