
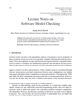
Safety-critical situations inevitably require human intervention, thereby, exposing personnel to formidable risks. This encompasses various domains, such as bomb disposal, emergency response, and hazardous material handling, all underlining the paramount importance of personnel safety. This research is dedicated to the development of a wireless robotic hand that replicates predefined gestures, facilitated by image processing algorithms. The hand gesture classifier attains an accuracy rate of 94.67% in identifying and distinguishing gestures. The implementation of hand gesture robotic hand emulators in safety-critical scenarios emerges as a pivotal strategy to potentially replace human participation. This substitution substantially mitigates risks for personnel. Moreover, it stands to enhance safety standards by virtue of its automated and controlled operation, thus minimizing the vulnerability associated with manual intervention. Fundamentally, this study acts as empirical validation of the substantial transformative capabilities inherent in robotics. This has the potential to completely reshape how dangerous tasks are handled in various industries which has a direct impact on keeping people safe and protecting valuable lives. Even though this research investigates a constrained selection of hand gestures and operates within a time limit for emulation, it establishes the fundamental basis for future progress in human-robot interaction and the realm of gesture recognition.