
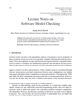
Automatic Traffic Sign Detection and Recognition (ATDR) system has been expanded and implemented partly in Intelligent Transportation System (ITS) that is actively used today. As traffic congestion increases, the manufacturing industry may have made and installed the ATDR systems in various types of vehicles, including cars, light commercial vehicles, and heavy trucks that act as driver assistance systems. ATDR system not only helps to minimize the number of traffic accidents but also supports road users through legal and compliant guidance and providing all traffic information, so that road users can be more attentive and solve them immediately in a short duration of time. On the point of the safety of the road users and others will be protected throughout a critical situation with identify the driving scene. In this paper, a deep-architectural neural networks, which is the convolutional neural network (CNN/ConvNet) model are chosen to expand in the ATDR system. With its excellent ability to train, research, and organize data, CNN is becoming one of the most widely used machine learning algorithms in key tasks such as prediction and classification. First, various open source of deep learning libraries will be studied. The library has been tested using the Malaysian Traffic Sign (MTS) dataset, which contains 32891 labelled images with real-world signs. Then the combination of both designed detection model and the CNN classification model will be trained under some parameter settings. Finally, the proposed system will detect and recognize the different types of traffic sign images in real-time display and Graphical User Interface (GUI). The achievable test accuracy is exceeded by 98% on a total of 43 different classes of MTS data that is obtained and for all study cases.