
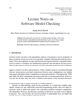
Location-based systems require rich geospatial data in emergency and crisis-related situations (e.g. earthquakes, floods, terrorist attacks, car accidents or pandemics) for the geolocation of not only a given incident but also the affected places and people in need of immediate help, which could potentially save lives and prevent further damage to urban or environmental areas. Given the sparsity of geotagged tweets, geospatial data must be obtained from the locative references mentioned in textual data such as tweets. In this context, we introduce nLORE (neural LOcative Reference Extractor), a deep-learning system that serves to detect locative references in English tweets by making use of the linguistic knowledge provided by LORE. nLORE, which captures fine-grained complex locative references of any type, outperforms not only LORE, but also well-known general-purpose or domain-specific off-the-shelf entity-recognizer systems, both qualitatively and quantitatively. However, LORE shows much better runtime efficiency, which is especially important in emergency-based and crisis-related scenarios that demand quick intervention to send first responders to affected areas and people. This highlights the often undervalued yet very important role of rule-based models in natural language processing for real-life and real-time scenarios.