
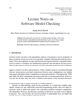
Predictions on product quality are essential for yield optimization, and cost effective manufacturing. Thus, intelligent sensory systems are applied for process monitoring, and control in numerous industries, e.g., paper-, steel-, or microelectronic industries. A plethora of effective methods, and techniques for the underlying large, and high dimensional problems have been developed, and applied. The goal of this work is to adapt, and to advance the existing wealth of experience to the so far scarcely covered field of polymer production. For this aim, an approach to a new system for rapid and reliable process analysis based on state of the art methods has been developed in this work and statistically meaningful sample sets have been extracted from different process levels. A clustering, classification approach was used on data sets of different batches. In a stepwise reduction of the sensory data selection from the complete 220 to 11 selected process sensory measurement points a predictive model was constructed which meets the industrial specifications of forecasting batch quality for minimizing defective goods by 5%, and raising the yield.