
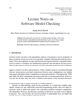
The fuzzy models have shown to be ambiguous and characteristically primitive in nature when applied for data analysis problems in most settings. This is owing to the fact that with fuzzy models, it may not be practically possible to extract meaningful information about the underlying process elements when confronted with datasets that are unstructured in nature. To this end, the work in this paper demonstrates that it is possible to extract abstract knowledge and improve the information values of such type of models to a greater level by carefully integrating and tuning the semantics metrics that the fuzzy models lack. Theoretically, the work introduces a semantic fuzzy mining approach that is particularly focused on making use of the fuzzy logic and theories to represent the imprecise and uncertain (unstructured) data about the different domain processes, and then presents the resulting models in a format that allows one to analyse the available datasets based on concepts rather than the tags or labels in the events logs about the processes in question. In other words, this paper adopts the fuzzy logic which permits a proposal to be in another state as true or false, and in turn, evaluates the outcomes of the method using a series of case study experimentation and its comparison against the other benchmark algorithms used for process mining. The result shows that it is possible to determine through the classification process (e.g. using a classifier/reasoner) the presence of different patterns or traces that can be found within the discovered models, as well as the relationships the different process elements share amongst themselves within the knowledge-base. Ideally, the method is described as a fusion theory which integrates the fuzzy model with other tools or method, thus, supports a hybrid intelligent system.