
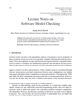
Background: electronic prescription is shown to have many benefits in terms of reducing medication errors, improving patient safety, productivity, and resource management, but it may cause new errors and physician frustration if not designed and implemented properly. Improving usability and user-centered design is essential for physicians' adoption.
Objectives: To enhance the efficiency of the e-prescribing system by reducing the risk of inappropriate selection of the medication and also to reduce the prescribing time and effort to reach the desired drug.
Methods: Important data fields for predicting medications were determined through interviews with pharmacists. Among those, fields which were available in a claims dataset of 16 million prescriptions were extracted and were used to develop a neural network model to be used by a recommender system that displays the most probable medications on top of the drop-down list in the e-prescription application.
Results: Offline and field evaluations both showed that this model could improve performance.
Conclusion: smart recommenders systems can improve e-prescription usability, safety, and enhanced physicians' adoption.