
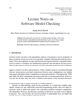
Background: In a database of electronic health records, the amount of available information varies widely between patients. In a real-time prediction scenario, a machine learning model may receive limited information for some patients.
Objectives: Our aim was to evaluate the influence of missing data on real-time prediction of delirium, and detect changes in prediction performance when training separate models for patients with missing data.
Methods: We compared a model trained specifically on data with missing values to the currently implemented model predicting delirium. Also, we simulated five test data sets with different amount of missing data and compared the prediction results to the prediction on complete data set when using the same model.
Results: For patients with missing laboratory and nursing assessment data, a model trained especially for this scenario performed significantly better than the implemented model. The combination of procedure data and demographic data achieved the closest results to a prediction with a complete data set.
Conclusion: An ongoing evaluation of real-time prediction is indispensable. Additional models adapted to the information available might improve prediction performance.