
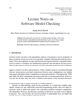
Human gesture recognition has assumed a capital role in industrial applications, such as Human-Machine Interaction. We propose an approach for segmentation and classification of dynamic gestures based on a set of handcrafted features, which are drawn from the skeleton data provided by the Kinect sensor. The module for gesture detection relies on a feedforward neural network which performs framewise binary classification. The method for gesture recognition applies a sliding window, which extracts information from both the spatial and temporal dimensions. Then we combine windows of varying durations to get a multi-temporal scale approach and an additional gain in performance. Encouraged by the recent success of Recurrent Neural Networks for time series domains, we also propose a method for simultaneous gesture segmentation and classification based on the bidirectional Long Short-Term Memory cells, which have shown ability for learning the temporal relationships on long temporal scales. We evaluate all the different approaches on the dataset published for the ChaLearn Looking at People Challenge 2014. The most effective method achieves a Jaccard index of 0.75, which suggests a performance almost on pair with that presented by the state-of-the-art techniques. At the end, the recognized gestures are used to interact with a collaborative robot.