
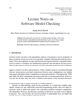
This work addresses active learning for multi-class classification. Active learning algorithms optimize classifier performance by successively selecting the most beneficial instances from a pool of unlabeled instances to be labeled by an oracle. In this work, we study the influence of the following factors for active learning: (1) an instance's impact, (2) its posterior, and (3) the reliability of this posterior. To do so, we propose a new decision-theoretic approach, called multi-class probabilistic active learning (McPAL). Building on a probabilistic active learning framework, our approach is non-myopic, fast, and optimizes a performance measure (like accuracy) directly. Considering all influence factors, McPAL determines the expected gain in performance to compare the usefulness of instances. For this purpose, it calculates the density weighted expectation over the true posterior and over all possible labeling combinations in a closed-form solution. Thus, in contrast to other multi-class algorithms, it considers the posterior's reliability which improved the performance. In our experimental evaluation, we show that the combination of the selected influence factors works best and that McPAL is superior in comparison to various other multi-class active learning algorithms on six datasets.