
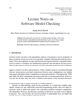
Observational ergonomic evaluation methods have inherent subjectivity. Observers’ assessment results might differ even with the same dataset. While motion capture (MOCAP) systems have improved the speed and the accuracy of motion-data gathering, the algorithms used to compute assessments seem to rely on predefined conditions to perform them. Moreover, the authoring of these conditions is not always clear. Making use of artificial intelligence (AI), along with MOCAP systems, computerized ergonomic assessments can become more alike to human observation and improve over time, given proper training datasets. AI can assist ergonomic experts with posture detection, useful when using methods that require posture definition, such as Ovako Working Posture Assessment System (OWAS). This study aims to prove the usefulness of an AI model when performing ergonomic assessments and to prove the benefits of having a specialized database for current and future AI training. Several algorithms are trained, using Xsens MVN MOCAP datasets, and their performance within a use case is compared. AI algorithms can provide accurate posture predictions. The developed approach aspires to provide with guidelines to perform AI-assisted ergonomic assessment based on observation of multiple workers.