
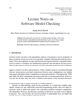
Dividing patients into similar groups plays a significant role in implementing personalized care. Clinicians and researchers have been applying patient grouping techniques in disease phenotyping, risk stratification, and personalized medicine. However, the current approaches are either based on pure domain knowledge where the underlying patient similarity cannot be precisely quantified, or based on unsupervised clustering techniques which completely ignore the clinical context of measuring patient similarity. In the study, we propose an outcome-driven approach to identify clinically similar patients which are grouped together as a precision cohort. The approach quantitatively measures the similarity between patients in terms of a particular clinical outcome of interest, thus patients who have a similar clinical outcome tend to be grouped into the same group. We demonstrate the effectiveness of the approach in a real-world case study: from an atrial fibrillation patient cohort that is usually considered to be at high risk for ischemic stroke (IS), according to current clinical guidelines. Our approach successfully identified a precision cohort of patients with truly low risk of IS.