
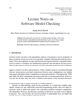
Surgical robotic systems and virtual reality simulators have introduced an unprecedented precision of measurement for both tool-tissue and toolsurgeon interaction; thus holding promise for more objective analyses of surgical skill. Integrative or averaged metrics such as path length, timeto- task, success/failure percentages, etc., have often been employed towards this end but these fail to address the processes associated with a surgical task as a dynamic phenomena. Stochastic tools such as Markov modeling using a `white-box’ approach have proven amenable to this type of analysis. While such an approach reveals the internal structure of the of the surgical task as a process, it requires a task decomposition based on expert knowledge, which may result in a relatively large/complex model. In this work, a `black box’ approach is developed with generalized cross-procedural applications., the model is characterized by a compact topology, abstract state definitions, and optimized codebook size. Data sets of isolated tasks were extracted from the Blue DRAGON database consisting of 30 surgical subjects stratified into six training levels. Vector quantization (VQ) was employed on the entire database, thus synthesizing a lexicon of discrete, task-independent surgical tool/tissue interactions. VQ has successfully established a dictionary of 63 surgical code words and displayed non-temporal skill discrimination. VQ allows for a more cross-procedural analysis without relying on a thorough study of the procedure, links the results of the black-box approach to observable phenomena, and reduces the computational cost of the analysis by discretizing a complex, continuous data space.